Unlocking the Power of Data Annotation Tools and Platforms
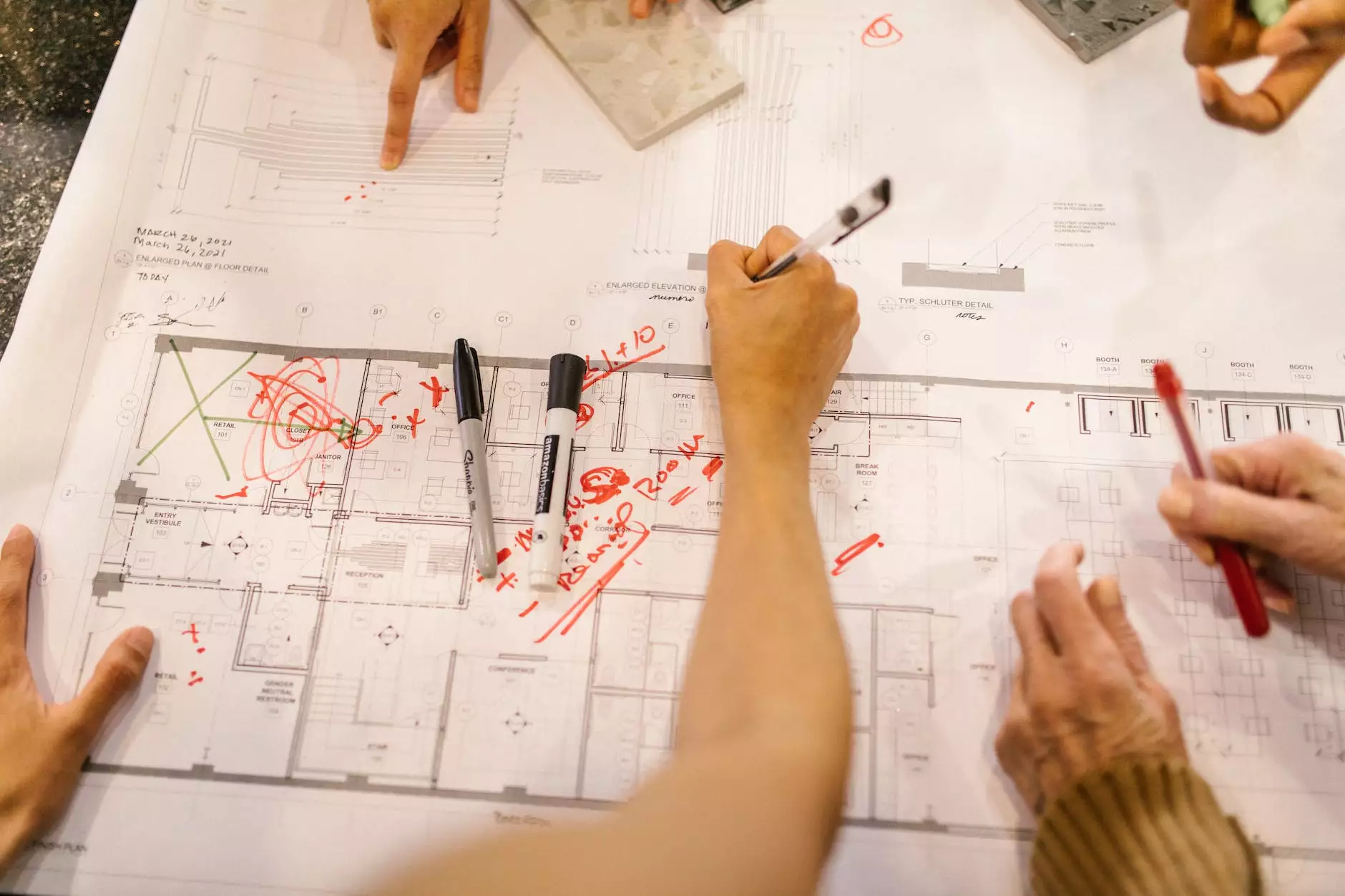
In the rapidly evolving landscape of artificial intelligence (AI) and machine learning, data acts as the cornerstone for developing effective models. However, raw data alone is insufficient for building high-performing AI systems. This is where the significance of data annotation tools and data annotation platforms comes into play, shaping the future of various industries. Whether you are an AI research lab, a budding startup, or an established enterprise, understanding and leveraging these solutions can lead to remarkable advancements in your projects.
Understanding Data Annotation
Data annotation refers to the process of labeling or tagging data to improve its quality for machine learning algorithms. The quality of labeled data directly impacts the performance of AI models; therefore, proper annotation is crucial. In simple terms, annotated data provides the context that machines need to understand and learn from the vast amount of information available.
The Significance of Data Annotation in AI
Data annotation plays a pivotal role in several domains, including:
- Computer Vision: Annotating images and videos with bounding boxes, labels, or segmentations allow computer vision models to detect objects and comprehend visual contexts.
- NLP (Natural Language Processing): Text data annotation includes identifying entities, sentiments, and categories, enabling language models to understand context and intent.
- Healthcare: Annotated medical images or patient records lead to better diagnosis predictions and treatment recommendations.
- Autonomous Vehicles: Annotating sensor data is critical for the safe navigation and operation of self-driving cars.
The Role of Data Annotation Tools
Data annotation tools offer automated or semi-automated solutions to streamline and enhance the annotation process. By facilitating faster and more efficient data labeling, these tools empower teams to focus on refining their AI models rather than getting bogged down by menial tasks.
Key Features of Data Annotation Tools
When selecting a data annotation tool, there are several essential features to consider:
- User-Friendly Interface: A straightforward interface ensures that both technical and non-technical users can annotate data effectively.
- Collaboration Capabilities: Real-time collaboration features enable multiple users to work on a project simultaneously, promoting seamless workflow.
- Customization Options: Tools should allow customization of annotation types to suit specific project needs, whether it's text, images, or videos.
- Quality Assurance: Built-in quality control measures help maintain high data accuracy and consistency through review systems and feedback loops.
- Integration Flexibility: Data annotation platforms should easily integrate with existing AI pipelines or tools, promoting efficiency.
Advantages of Using Data Annotation Platforms
Data annotation platforms provide comprehensive solutions, often offering cloud-based systems that can handle large-scale annotation projects. Here are several advantages associated with using dedicated platforms:
Scalability
Data annotation platforms are designed to handle projects of all sizes. Whether you're processing a few thousand data points or millions, robust platforms can scale to meet your demands without sacrificing quality.
Enhanced Productivity
By automating repetitive tasks and providing intuitive workflows, data annotation platforms significantly enhance productivity for teams. This allows data scientists and machine learning engineers to concentrate on more critical tasks.
Cost-Effectiveness
Investing in an efficient data annotation platform saves both time and resources. The cost associated with poor data quality far outweighs the initial investment in reliable annotation services.
Choosing the Right Data Annotation Solution
With various options available in the market, selecting the appropriate data annotation solution might seem daunting. However, following these structured steps can simplify your decision-making process:
- Define Project Requirements: Identify the type and volume of data you need to annotate, the required annotation styles, and the expected timeline.
- Evaluate Features: Compare features across different platforms to ensure they meet your technical requirements, focusing on usability, automation, and quality assurance.
- Check Reviews and Testimonials: Investigate user reviews and case studies to gauge the performance and reliability of each platform.
- Consider Pricing Models: Evaluate the pricing structures to find a solution that aligns with your budget while fulfilling project needs.
- Consult with Experts: If possible, engage with industry experts or consultants who can offer insights and recommendations tailored to your specific applications.
Implementing Data Annotation in Your Workflow
Once you have selected the appropriate data annotation tool or platform, it’s crucial to effectively integrate it into your workflow. Here’s how:
1. Training Your Team
Ensure your team is trained in the functionalities of the chosen tool. Offering adequate training will boost their efficiency and understanding of the annotation process.
2. Setting Clear Guidelines
Establishing clear guidelines for annotators will help in maintaining consistency across the entire dataset. Documenting styles, formats, and rules is essential to achieve high-quality results.
3. Conducting Regular Reviews
Incorporate regular reviews of annotated data to monitor quality. Employing several rounds of feedback will help catch errors early in the process.
4. Iterating on Your Process
Digital annotation is not static; continuously assessing and refining your approach based on feedback and results will lead to constant improvement in your data quality.
Real-World Applications of Data Annotation
Data annotation has proven to be invaluable across numerous industries. Here are some exemplary applications:
1. Retail and E-commerce
In retail, data annotation enables product recognition and recommendation systems. By correctly annotating product images and descriptions, companies can enhance customer experiences and drive sales.
2. Finance
Financial institutions utilize data annotation for fraud detection systems. Annotating transaction data helps identify patterns indicative of fraudulent behavior, thereby strengthening security measures.
3. Social Media
Social media platforms rely on data annotation for content moderation, sentiment analysis, and targeted advertising. Annotated texts and images enhance user engagement by delivering relevant content.
4. Energy Management
Energy companies use data annotation for monitoring and predictive maintenance. Annotating sensor data from machinery helps teams understand performance and anticipate failures, optimizing operational efficiency.
Future Trends in Data Annotation
The field of data annotation is constantly evolving, as new technologies and methodologies emerge. Here are some future trends to watch for:
1. Automation and AI-Driven Annotation
As AI technology continues to advance, automated annotation tools are becoming increasingly common. These solutions can analyze and label data with minimal human intervention, speeding up the annotation process significantly.
2. Crowdsourced Annotation
Crowdsourcing is being employed to leverage the power of many individuals to annotate vast datasets quickly and accurately. This approach can significantly reduce costs and turnaround times.
3. Multi-Modal Annotation
With the rise of complex AI applications, there’s growing demand for multi-modal annotations that span various types of data formats, including text, images, videos, and audio.
4. Emphasis on Quality over Quantity
As AI models become more sophisticated, the focus on high-quality annotated data will become even more paramount. Companies will prioritize creating smaller datasets that are meticulously annotated over larger, less reliable datasets.
Conclusion
In summary, the significance of data annotation tools and data annotation platforms cannot be overstated in the era of AI and machine learning. By enhancing the quality of data, these solutions facilitate the creation of effective and reliable AI models. As the industry evolves, leveraging advanced annotation tools will be instrumental in staying competitive and innovating within your field.
To learn more about how effective data annotation tools can transform your AI projects, visit Keylabs today.
https://keylabs.ai/